Table of Contents
- Introduction to a Tradition: What is Perceived Message Effectiveness?
- Examples of Typical Message Testing Data
- When PME was “the” Metric to Use
- Sobering Recent Discoveries About PME
- Challenge 1: PME metrics are all intercorrelated, so why do we need to ask them all?
- Challenge 2: PME is correlated with AME, but not strongly enough to make confident decisions
- Challenge 3: PME could mislead us when choosing between candidates
- Summary: PME picks winning messages at just-better-than-chance levels
- Closing Thoughts
Introduction to a Tradition: What is Perceived Message Effectiveness?
Having been in the primary insights game for a few decades now, we’ve done a lot of message testing. In almost all cases, whether the study is qualitative or quantitative, we do some kind of task that compares a set of messages on a common metric to help us pick which messages will perform better in a real-life campaign. Most of the time researchers would do this using an anchored numeric or categorical scale, or several such scales, which might include concepts such as:
- Perceived message effectiveness (PME)
- Message persuasiveness
- Message believability
- Message relevance
- Message differentiation
For simplicity, we’ll use the acronym PME to refer to any of the above metrics, consistent with the scientific literature terminology.
Most message campaigns are trying to produce some kind of a change in one of the following endpoints.
- Attitude toward a product
- Beliefs about a product
- Behavior or intended behavior toward a product
The fundamental question is, does PME (or its cousins) have any systematic relationship with AME (actual message effectiveness)? We want the answer to be a resounding “yes!” But is it?
Examples of Typical Message Testing Data
To start our review, let’s take a look at the kind of outputs we generally observe with the data that you get from traditional PME metrics. In the first example, let’s imagine we evaluate two messages, G and T, on perceived message effectiveness (PME), which are shown in Table 1 (assumes a 1-7 anchored scale).
Table 1. Example Message Effectiveness Scores

Even if the differences between G and T were “statistically significant,” would you feel confident that Message T was really going to be the slam-dunk winner? We can make this even more uncomfortable by coming up with more fictitious-but-absolutely-realistic data that use a variety of perceptual metrics from the list above. Table 2 shows hypothetical data for a set of metrics (same type of 1-to-7 anchored scale). Let’s also assume that the first three measures are statistically significant (intent-to-prescribe measures are rarely significantly different in message testing).
Table 2. Example Message Evaluation Scores on Multiple PME-type Dimensions
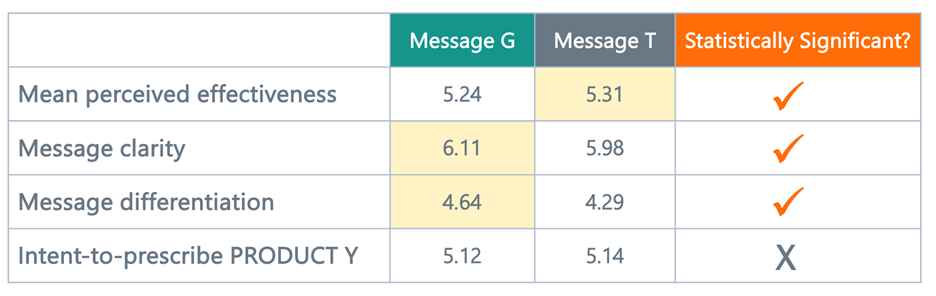
Now what? Message G has a “clear” edge on clarity and on differentiation. But Message T wins on effectiveness, and to some vanishing degree on intent-to-prescribe. Which is the winner? Which metric is more important? For primary research veterans, this scenario is likely to feel eerily familiar. PME is not giving us much clarity in this situation.
When PME was “the” Metric to Use
People who study messaging for a living as professional academics also use PME for predictive purposes. They do it for the same reasons we do: (1) It is easy and practical. (2) Conceptually it makes sense to ask these questions. (3) There’s a strong precedent for doing it this way. (4) In the day, there was some evidence that PME was “the metric.” A very famous study (regarded and described as a “classic” by the Journal of Advertising Research) published in 1991 found evidence that metrics that captured the same essential idea as message effectiveness (e.g., ad persuasiveness) were the best measures among a host of competing metrics at discriminating winning vs. losing TV advertisements tested in a range of markets. That conclusion was advanced by a substantive re-examination of the original findings published a few years later. There is probably more to this history, but the popularity of this work underscores why there is more than simple precedent to go on when researchers use PME-type measures.
Sobering Recent Discoveries About PME
Over time, and particularly since 2010, a very large number of studies have been conducted on message effectiveness both inside and outside of healthcare using these kinds of evaluation metrics. One really great thing about this accumulated research is that it has allowed behavioral scientists of various stripes to synthesize and integrate the findings from a diverse body of studies. Since the mid-2000s, several major meta-analyses have allowed us to draw some salient practical generalizations about the quality of PME-type data as they relate to AME. Rather than boring you with a complete explanation, it’s more fun to jump to the punchlines.
Challenge 1: PME metrics are all intercorrelated, so why do we need to ask them all?
One major research synthesis included illustrative examples of how PME-type measures (see list) are highly intercorrelated. In fact, if you did a factor analysis, they would all load on one component and display high internal consistency – in the range of ~0.95. That explains the fictitious-but-absolutely-realistic data shown in Tables 1 and 2. Stepping back for a moment, if we consider the largely heuristic-driven, gist-based way in which people tend to evaluate stimuli, this finding makes absolute sense. Participants don’t “feel” the distinction between “clarity” and “personal relevance” or “believability” and “effectiveness.” As marketers, it seems obvious and logical to look for these differences, so we are not questioning why researchers include them. But if they are unintentionally measuring the same thing (as the meta-analytic data suggest), we ought to ask whether forcing participants to give us answers for all these items is adding value to the research.
Challenge 2: PME is correlated with AME, but not strongly enough to make confident decisions
Another major finding was that, on average, PME was correlated with simultaneously-measured attitude changes at r=0.41. This seems pretty decent because we have all heard that “In psychology, everything correlates with everything at 0.3.” And in all seriousness, in many areas of science 0.41 would be a pretty whopping effect size. However, there are two problems. The first problem is that this means that PME accounts for roughly 17% of the total variance in attitude change. Given the vagaries of attitude measurement and the normally limited correlation between attitudes and emergent behaviors, this feels a little weak for business decision-making.
Challenge 3: PME could mislead us when choosing between candidates
Another problem is that if you are testing a bunch of messages, knowing that the general correlation between their earned PME scores and downstream attitude change is only 0.41 should be disquieting. Is that a big enough correlation to be confident that Message T’s slightly superior PME over Message G will actually translate to better downstream attitude change? What about Message G versus Message E? And Message E versus Message B? Somewhere in that matrix of pairwise comparisons, that low correlation will probably mean that PME will mislead us. To make this clearer, Table 3 illustrates how even relatively highly correlated PME-AME data could lead you to make bad choices. In this example, we have eight hypothetical messages (A to H), each of which gets a PME score on a 1-to-7 scale. If we were magically able to know the actual message effectiveness that would play out in the real world, a correlation of 0.41 would lead to a set of AME scores like the ones in the third column. In concept, we could simply rank the messages based on PME and then use the rankings to pick the winning message. Since PME and AME are correlated, we might expect this approach to produce the right outcome. If you simply picked the top message (H) based on PME, you would have chosen the fourth-best message in terms of actual real-world performance. In fact, despite moderately high correlation, none of the PME-AME ranks are the same for any single message.
Table 3. Illustrating the limitations of a PME-AME correlation using r = 0.41 as an example
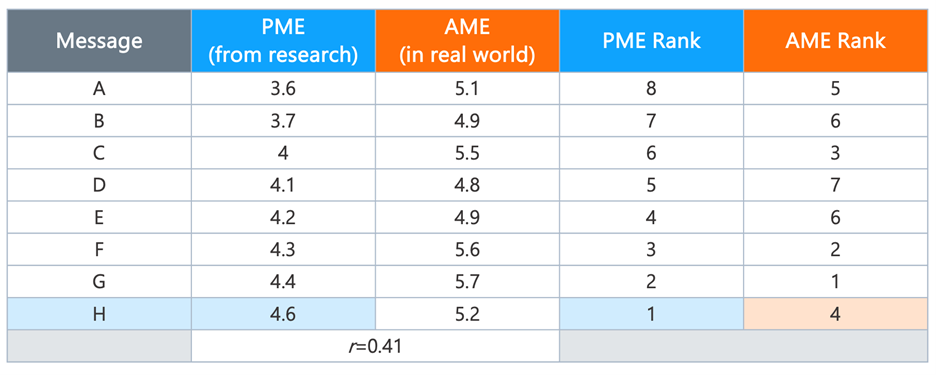
Summary: PME picks winning messages at just-better-than-chance levels
A meta-analysis, published in 2018, addresses the “correlation” problem above. This study looked at PME and AME scores at the level of pairs of messages. For example, if a study had three messages, they would compare the PME-AME performance for A to B, B to C and A to C. Then they aggregated these relationships across multiple studies. The reason for analyzing the PME-AME relationship between message pairs is that most marketers want to use PME data to select one message over another. So the analysis matched the way people actually want to use PME data in real life. The dependent measure was elegant: Because each study included independent assessments of PME and AME for all message pairs, the authors were able to evaluate the proportion of pairwise cases in which the PME score predicted the winning message (in terms of AME). If you simply picked one message from each pair at random (e.g., you flipped a coin), you would expect to predict the higher performing message 50% of the time. The researchers found that the higher-PME message predicted the higher-AME message 57.6% of the time. In plain language, that means that PME scores are just a whisker better than a coin toss at telling us which of two messages will do better at shaping customer outcomes in the wild. To put that differently, you would choose the wrong message a lot of the time.
Closing Thoughts
This summary is not intended to suggest that there is no value in using conventional PME-type measures. They can allow us to do things like TURF analysis and they offer conveniently structured dependent variables for use in testing bundles of messages using experimental designs. However, the limitations are real and relatively serious when it comes to selecting one message over another. Happily, newer and more diverse methods of message evaluation offer superior predictive insight relative to real-world performance, and based on the findings discussed above, we believe those options are worth exploring. This is a great example of how advances in understanding from accumulated data can really help us to improve the practice of marketing.
To learn more, contact us at info@euplexus.com.
About euPlexus
We are a team of life science insights veterans dedicated to amplifying life science marketing through evidence-based tools. One of our core values is to bring integrated, up-to-date perspectives on marketing-relevant science to our clients and the broader industry.
References
Dillard, J. P., Weber, K. M., & Vail, R. G. (2007). The relationship between the perceived and actual effectiveness of persuasive messages: A meta-analysis with implications for formative campaign research. Journal of Communication, 57(4), 613-631.
Haley, R. I., & Baldinger, A. L. (1991). The ARF copy research validity project. Journal of advertising research.
High, A. C., & Dillard, J. P. (2012). A review and meta-analysis of person-centered messages and social support outcomes. Communication Studies, 63(1), 99-118.
Nabi, R. L. (2018). On the value of perceived message effectiveness as a predictor of actual message effectiveness: An introduction. Journal of Communication, 68(5), 988-989.
Noar, S. M., Bell, T., Kelley, D., Barker, J., & Yzer, M. (2018). Perceived message effectiveness measures in tobacco education campaigns: A systematic review. Communication methods and measures, 12(4), 295-313.
O’Keefe, D. J. (2018). Message pretesting using assessments of expected or perceived persuasiveness: Evidence about diagnosticity of relative actual persuasiveness. Journal of Communication, 68(1), 120-142.
O’Keefe, D. J. (2020). Message pretesting using perceived persuasiveness measures: reconsidering the correlational evidence. Communication Methods and Measures, 14(1), 25-37. Rossiter, J. R., & Eagleson, G. (1994). Conclusions from the ARF’s copy research validity project. Journal of Advertising Research, 34(3), 19-33.