Table of Contents
- Overview
- What is the “Conjoint Family?”
- Why Do People Like the Conjoint Family of Methods?
- Do Conjoints Have Special Revelatory Powers?
- Three Fundamental Problems the Conjoint Family
- Are Conjoints More Accurate?
- The Problem of “Nonsense Effects”
- Data Quality & the Survey Experience
- A Life Science-Appropriate Solution: Self-Explicated Conjoint
- Wrap-Up and Take-Home Points
Overview
This article presents an evidence-based perspective on the life science industry’s reflexive use of what we call the “conjoint family” of experimental procedures for testing attribute importance and product profile variations within demand studies. First, we explain some of the basics of when, where, and why these designs are used. Next, we explain some misperceptions about them, and introduce three persistent and very serious problems with these designs. Finally, we present a viable replacement called the Self-Explicated Conjoint that is, frankly, superior on a range of business relevant dimensions and which enjoys strong support in the behavioral science literature. Throughout, we’ll bring in the best data we can find from contemporary science to help us make the case.
What is the Conjoint Family?
We use the shorthand phrase “conjoint family” when we want to make reference to the range of techniques that use experimental designs to explore the relative importance of product attributes as they relate to customer preference and decision-making. To take this one level deeper, these methods involve (1) building/testing a large number of product variations, (2) an experimental design where each participant only sees a fraction of the variations, and (3) regression-based determination of the relative importance of attributes on some outcome variable.1 If you meet these criteria, you are in “the family,” which encompasses classical conjoint, discrete choice modeling (DCM), adaptive conjoint, rank-based conjoint and can even be stretched (on occasion) to include MaxDiff. In large part, these techniques originally came to us from mathematical psychology and marketing science, where they were developed based upon the premise that the value of a product to a consumer could be modeled as the sum of the value of all its features or benefits. But very similar ideas have also been around in economics since the 1960s, where product value was first conceptualized as being a function of the individual product features. In the decades since they were first introduced these techniques have become extremely popular, including in the life science arena. In fact, according to some sources, they are far more popular now than when they were first introduced.2
These techniques allow you to “dissect” a product down to its component parts and quantify exactly how much its different features matter to customers. This knowledge is critical for marketers who have to devise compelling stories about their brands, and to product developers who have to build the next thing customers will buy. And for us in the life science space, there are plenty of situations when we will need to do this. However, one reality is that if you are going to isolate the value of product attributes that can vary (e.g., you may have 3 or 4 or 5 levels of efficacy to test), you will need to test a large number of different versions of your product. In addition to the benefits of understanding the worth of different product features, these studies also are helpful in managing uncertainty around our products’ performance. Researchers, forecasters and marketers often use these techniques in the context of demand work because we don’t always know how our products will turn out, and we want to be able to understand how customers will value them under different circumstances. The process of setting up the range of performance variations has its own vocabulary, which is framed out in Figure 1 below.
Figure 1. Base Case Product Profiles, Attributes and Levels in TPP Testing
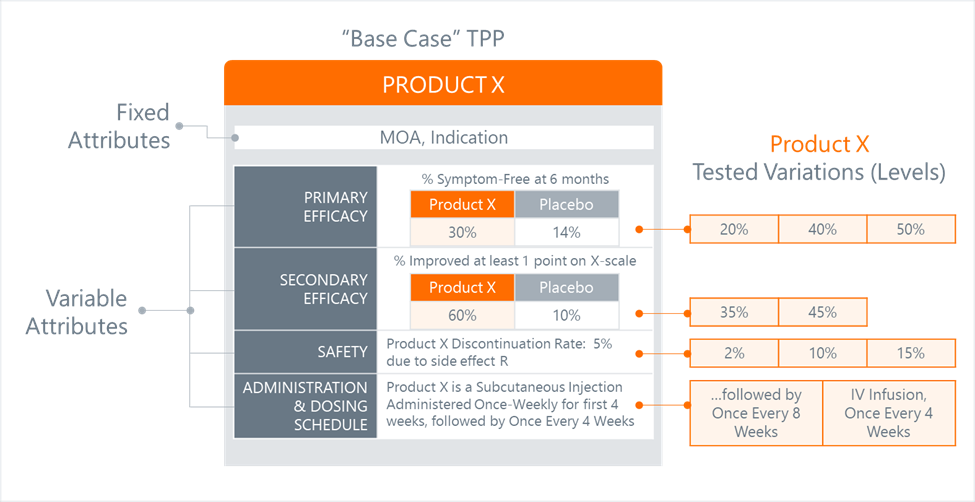
Normally, you would use one of the conjoint family methods when you have so many TPP variations that you cannot possibly show all of them to respondents. To deal with this problem, we resort to experimental design and “fractionation” of our sample. The basic idea is that you define a set of TPPs by concatenating a bunch of different attributes and levels. From here, software is used to determine the most efficient way to organize and test the large set of TPPs. The experimenter will be looking to make sure that some minimum number of participants sees and responds to each TPP variation. For example, some percentage of participants will see, variations 4, 21, 17, 2, 20, 12, 7 and 24, while some other percentage will see variations 23, 21, 5, 3, 13, 14, 10, and 2. The goal is to get sufficient data for each variation to allow the modeler to estimate the demand for all possible variations. This process is summarized in Figure 2 below.A
Figure 2. Simplified View of How Fractionated Designs Work
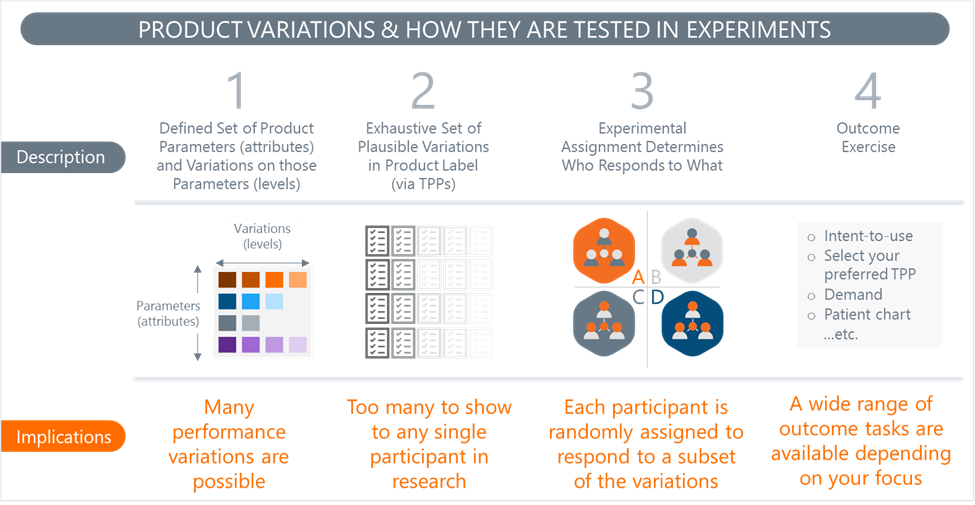
A We recommend Vithala Rao’s book, Applied Conjoint Analysis, and suggest this as a reasonable point-of-entry if you want to deepen your exposure and understanding. See References for the exact citation.
Why Do People Like the “Conjoint Family” of Methods?
On the face of it, there’s a lot to like about these methods. For me, the benefits generally fall into four categories.
- Ability to Test Lots of Stuff:
- The main benefit for using them is that the reliance on experimental designs allow you to test a very large number of product variations without (ostensibly) over-taxing your participants. As we will see below, there are plenty of situations where this capability is really useful for a variety of stakeholders.
- The “Magic” Power of Derived Importance:
- Second, because we have a cultural bias to not trust self-stated importance or intentions in this industry, we get the superficial comfort of knowing that our attribute importance ratings are “derived” – meaning that they come from regression rather than the minds of our treaters and patients. It just feels like derived importance must be better than self-stated importance. We’ll come back to this.
- The Complexity = Quality Heuristic:
- Many of us (me included) like fancy methods. Conjoint/DCM studies require the use of experimental designs and advanced analytic approaches, and often depend upon complex software. The heuristic that says “complexity/sophistication = quality” is well documented in research on information quality assessment3, and I believe it is a key reason why some people feel comfortable using these approaches.
- The Institutional Imperative:
- I love this term, which I stole from Warren Buffet and the late, great Charlie Munger. The institutional imperative is simply the normal tendency for organizations to imitate each other, just as individual humans do. The use of the “conjoint family” is now widely accepted, in part because people learned that other organizations were doing it in their market research. Another way to say this is that, in life science, as an insights professional, you can’t get in trouble for using a conjoint or a DCM. They are accepted as good, vetted methods.
And, as we alluded to a moment ago, we use these tools in a range of scenarios and for a range of purposes, including4 :
- To inform investment decisions by understanding what kinds of product features are appealing to customers. Knowing this can help focus product evaluation and prioritization.
- Exploring which product features and thresholds of performance will be most appealing to customers during early stages of product development with owned assets. This can inform clinical trial design, dosing, endpoint selection and route of administration decisions at stages where flexibility still exists.
- For later-stage assets, these kinds of studies can inform marketing strategy (as in, which features do we emphasize in our advertising and detailing?), label negotiations (as in, what claims will be most valuable to have in your PI?), and forecasting (as in, what happens if we achieve/fail to achieve some particular degree of performance?).
All of these needs have some commonalities but come from very different commercial points of view. And to be clear, we agree that the conjoint family of methods represents one very good approach to addressing these problems.
Do Conjoints Have Special Revelatory Powers?
One issue that needs some clearing up is persistent belief that the conjoint family has the power to reveal things about customer preference and the value placed on product attributes that direct inquiry simply cannot produce. I have encountered this viewpoint either explicitly or tacitly in many conversations over the years. And there is a specific connotation that goes along for the ride, which is: These exercises can show us hidden aspects of customer psychology that are not accessible to the customers themselves.5 To be honest, this is mostly mythology and I think the misperception is prevalent enough to warrant some debunking.
Let’s start with some terminology. Two expressions are used commonly to connote the special powers of conjoint designs. The first is “revealed preference,” which is juxtaposed with the ostensibly more pedestrian “stated preference.” The second is “derived importance,” which is juxtaposed with the presumably inferior approach of capturing “stated importance.” The terms have different origins and refer to different endpoints, but I hear them used almost interchangeably in conjunction with experimentally-based methods from the conjoint family. Revealed preference comes to us from economics, where it refers to an important phenomenon. From the mid-1960s onward, many economists have studied peoples’ willingness to pay for public goods – and the consistent finding was that what people said they would pay in hypothetical exercises was considerably higher than what they would actually pay in real-life situations. The term revealed preference refers to these real-life observations. When economists refer to stated preference, they do not distinguish between straightforward stated preference exercises and experimentally based exercises, such as conjoints. They recognize that both types of exercises are measuring stated preference based on a hypothetical exercise. In other words, whether your study involves a fancy experiment or a simple self-stated task, you are getting a measure of stated preference in either case. Revealed preference is what you see when your product actually comes to market, not what you get from a PMR exercise. On the other hand, “derived importance” is an appropriate term to use with conjoint, as the conjoint family absolutely does give you derived estimate of relative attribute importance. These estimates are “derived” in the sense of being modeled via regression. This means that the relative strength of each attribute (and their corresponding levels) is determined mathematically based on variations in the product permutations that are shown in the experimental exercise.
The key question is, do conjoints produce better data about attribute importance compared with conventional rating-based exercises or open-ended explorations of the same topic? The reality is that there is not a huge amount of experimental evidence comparing the ratings of attribute importance that you get from self-stated exercises (such as attribute list rankings, scalar ratings of importance or chip/point allocations) compared with importance scores from “derived” exercises.6 But a 2007 research synthesis from the Journal of Business Research offers what I suspect is the best, most nuanced view of the available data.7 The authors evaluate ten widely-used approaches to attribute evaluation, including various types of conjoint-style designs, free association exercises and self-stated/direct measure exercise (such as importance ratings, point allocations and ranking exercises). At a basic level, they note that the correlations between the ten different types of attribute importance tasks are generally pretty low (less than r=0.35 in virtually all cases). And they cite several studies that show low correlations between conjoint-family exercises and attribute rating/ranking exercises (in the range of r=0.2 to r=0.3 depending on the task pair). This certainly suggests that, at a minimum, conjoint-type exercises are not getting at exactly the same information that direct rating gives us. But is one better than the other?
To answer this, the authors synthesize findings from 34 published studies comparing various attribute importance evaluations against real-world outcomes, such as which product is actually selected, or which ones are most likely to be purchased in the future. Their findings are summarized in Table 1 below. As you can see, the correlations between the two types of tasks and the downstream outcomes are virtually identical, and very strong in both cases.
Table 1. How Well do Conjoint-Family and Self-Stated Tests of Attribute Importance Correlate to Real-World Outcomes?

So how can they be well-correlated with outcomes but weakly correlated with each other? To square this circle, authors elegantly hypothesize that the different types of attribute importance tasks are actually measuring different aspects of the concept of importance. Specifically, they argue that:
- Self-stated attribute importance is mostly measuring Relevance: The extent to which specific attributes are aligned to felt need and personal values.
- Conjoint-family methods are mostly measuring Determinance: The extent to which specific attributes drive choice/decision-making in the moment.
Both these “lenses” on attribute importance are obviously valuable to marketing, but they are somewhat different. The authors conclude that the choice of your attribute evaluation task should be driven by which view is most important to your brand. They also note the value of taking a multi-method approach to attribute importance.
The bottom line is that conjoint-family methods appear to be no better and no worse than simple direct-rating tasks when it comes to predicting real world outcomes. Some people might see this summary as heretical – after all, beliefs about the magical revelatory power of conjoint designs have been around at least as long as I have been in the industry. All I am asking is for people to consider the scientific view on this issue.
Three Fundamental Problems with the “Conjoint Family”
A friend and former colleague once told me that I have tended to be “a little too hard on conjoint,” and no doubt you have already guessed that I am not a huge fan of this family of methods in my own practice as a researcher. To be clear, I do believe they have value, but I think that value is highly circumscribed when it comes to the life science industry specifically. My basic position is that we are over-using them and doing so in scenarios that do not support them, and the place that is probably most problematic is in the practice of demand research.
There are three fundamental problems with these approaches as they pertain to the life science arena and to demand studies in particular. Each of these issues could be its own article, but I’m going to try to give you a basic evidence-based perspective for each one.
- Problem 1: Conjoints are NOT more accurate than direct measures of intended behavior when it comes to predicting actual customer preference.
- Problem 2: Achievable samples in the life science arena coupled with normally tiny effect sizes associated with marketing content create a perfect situation for what we call “Nonsense Findings” (aka “reversals”) when we use fractionated experimental designs.
- Problem 3: Conjoints require a ton of cognitive “lifting” and make very large assumptions about how customers read, interpret and assimilate information in our TPPs.
Any one of these problems might not be enough to tilt me away from these techniques, but the confluence certainly does. Let’s unpack each one in a bit of detail.
Are Conjoints More Accurate?
In 2020, a group of researchers published a very practical meta-analysis on the topic of hypothetical bias, a term that refers to the tendency for humans to overstate their interest in or willingness to pay for products.8 That tendency is especially relevant in the context of demand studies, as we discuss in our article entitled “How Biased Are Demand Estimates?”. This paper, which represents the largest and most applicable synthesis of the estimation bias phenomenon that I am aware of, also did a specific sub-analysis comparing the degree of inflationary bias associated with direct measurement exercises compared with choice-based exercises from the conjoint family. They did this because, for years, choice-based exercises have been put forward as uniformly better than standard hypothetical estimation methods that ask customers to estimate their willingness-to-pay with a direct question.
Contrary to what some might expect, the meta-analysis showed that choice-based methods systematically led to larger bias – specifically an increase of +10.8% in total bias. In other words, conjoint and DCM-style procedures actually increased the degree of inflationary bias in hypothetical vs. real-world comparisons. Moreover, this problem seemed to persist no matter how the authors cut the data. We’ve reproduced this important finding in Table 2 below. This synthesis reflects 77 studies and 115 individual effect sizes, and represents more than 20,000 participants, so it is reasonably robust.
Table 2. Persistently Higher Bias with Choice-Based Exercises Compared with Direct Assessment
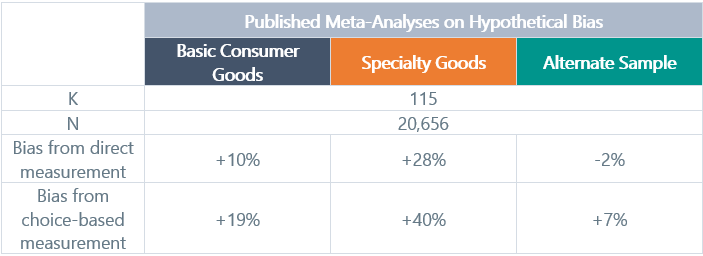
The authors devoted a lot of real estate to these findings in their paper, and they did that because they knew that these findings went against prevailing wisdom. The fact that this increased inflationary bias shows up in roughly the same proportion for a variety of sub-analyses further undergirds our confidence in the findings. To be completely clear, these data are hardly some death knell for the conjoint family – and that isn’t the point of showing them. What they do tell us is the prevailing belief that experimentally based exercises in the conjoint family DO NOT produce more accurate estimates from research participants compared with simpler, direct-measurement approaches. Based on this, I think it’s unreasonable to expect a different finding in a life science demand study, where we are using hypothetical willingness-to-prescribe to estimate future behavior.
Another meta-analysis published in 2013 specifically set out to demonstrate that the proliferation of the conjoint family of methods over the last fifty years would inevitably lead to improved evidence of predictive validity across time.9 Interestingly, they found the opposite. This synthesis included 2,093 data sets from commercial marketing applications of conjoint research captured between 1996 and 2011, featuring studies from a range of industries including life science, and including a range of different types of conjoints representing different members of the conjoint family. The net conclusion is that the external validity of these applied studies of conjoint techniques deteriorated over time. There’s nothing damning about this finding either – it simply underscores that the downstream validity of these methods has been and remains an issue. Overall, when we look at these streams of evidence, I think it is reasonable to conclude that conjoints do not produce more accurate pictures of the real world compared with direct, self-stated methods.
A Quick Sidebar About Meta-Analysis:
This may be an opportune moment to say something about the importance of meta-analysis. I was once in a conversation with another methodologist who was a strong exponent of conjoint designs and felt they should be used wherever possible in life science demand assessment. During my attempts to persuade him that there were real evidence-based problems with the method and that I could adduce academic evidence to support my view, he offered a rejoinder that was something to the effect of the following: “Well, pick any phenomenon and I can find you a study that supports it and another study that refutes it.” I think many people feel this way about behavioral science – the evidence is all over the place.
The truth is that my colleague was absolutely right, which is why meta-analysis is such an important tool for behavioral researchers. If I have one study that says “A is better than B” and a second, similar study that says “B is better than A,” I cannot reasonably prefer one option over the other. But if I have 20 studies of similar quality and sample size that all indicate “A is better than B,” and only 5 studies that say “B is better than A,” I would be foolish to make a strong declaration about the superiority of option B. When experimental evidence accumulates over time and a meta-analysis offers a clear synthesis of those data, it really is telling us something, and it is no accident that a large, well-done meta-analysis is often used as a gold standard for establishing guidelines in clinical research.
The Problem of “Nonsense Effects”
The conjoint family of techniques also runs into basic statistical problems when deployed in life science settings. When people do conjoint analysis in consumer research, their sample sizes are typically in the range of about n=1,000 on the low side and >n=2,000 on the high side. These figures come from the above-referenced 2020 literature review, so they ought to be reasonably up to date. There are two questions I will pose based on this. When was the last time you did a conjoint study in healthcare that had a sample greater than n=200? I suspect it has been a while. Second question: Why would you need that much sample to begin with? The answer to the second question can be found in another life science data phenomenon: marketing effect sizes are generally quite small, and this is especially true in life science marketing. We demonstrated this quite clearly in one of our science posts entitled, “Why We Can’t Expect the Same Level of Impact from Healthcare Messaging vs. Other Industries”, where we cited an enormous 2022 meta-analysis showing that effect sizes in healthcare are about half the size of effects from consumer marketing, generally about r=0.05 after a single exposure to some kind of stimulus, such as a TPP or concept. The empirical reality is that, in life science we are normally in the situation of having to detect smaller (i.e., harder to see) effects from our content testing while working with sample sizes that might be 1/10th to 1/5th the size of the samples in consumer land. A lovely paper on practical power analysis in Biochemia Medica provides a handy one-stop assessment for roughly estimating the sample size needed to detect specific effect sizes. Using their framework, I found that based on the typical effect mentioned above, even achieving a nominally acceptable level of statistical power would require somewhere in the neighborhood of n=2,000 for a between-subjects design such as a blocked conjoint.10 That’s obviously not realistic. And if you disbelieve me, please feel free to test my assertion using available power analysis software such as G*Power.B
When you put small effect sizes together with low power, it is a recipe for what I call “nonsense findings.” Here’s an example of what I mean. In Table 3, below, we have two experimental groups where different subsets of our sample are exposed to two versions of a TPP. Let’s assume that these came from a DCM-based design that uses sample blocking, such that if I am assigned to see TPP version A, you are assigned to B, and neither of us sees what the other sees. Take a quick look at the product characteristics. Which one looks better to you? Now look at the demand. Does it seem reasonable to you? This is a simple and modest example of what we mean by nonsense findings. Now imagine that you have a huge range of TPP variations. What are the chances that you will see patterns in the data that cannot be explained logically? The answer can be estimated safely at ~100%. It is also no exaggeration to say that the chances of this are sharply augmented by the fact that in life science we can rarely achieve sample sizes that can support these enormous designs.C
It is also possible for simple sampling error to contribute to the likelihood of nonsense findings.
Table 3. Illustrating the “Nonsense Findings” that Can Arise from Fractionated Designs
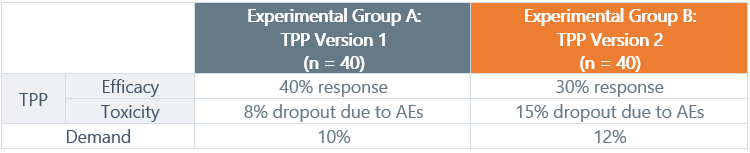
When confronted with this kind of issue, the options for the researcher are never particularly ideal. And you can imagine that the temptation to simply “make the problem go away” is substantial, because there is simply no coherent way to explain such findings to a marketing team. If you have ever had the “privilege” of presenting this kind of data, you’ll know what I’m talking about.
B G*Power is a great piece of freeware that we highly recommend. http://www.gpower.hhu.de. If you are interested in doing your own power analysis on the above-referenced average healthcare marketing effect size, I recommend converting it to Cohen’s d, which would translate in this case to d=0.10.
C For a more detailed discussion of this phenomenon, as it plays out in larger designs, see Choi (2005) in the reference list.
Data Quality & the Survey Experience
The final problem with these designs has to do with basic data quality stemming from cognitive issues. I am going to make the assumption that anyone reading this post has some knowledge of the effects of cognitive fatigue on survey data quality, which is now heavily documented in academic synthesis of survey research methods.11 Common effects include deterioration in volume and specificity of open-end responding, reduced variation in response option use and increased rates of intentional question-skipping. Not surprisingly, textbooks that teach students how to use conjoint methods also admonish us to be careful about data quality. The reasons for this concern are straightforward and basically amount to the following issues that arise when we ask participants to review more than a handful of TPPs.12
- Participants often do not read carefully.
- Participants become fatigued and their attention begins to fade.
- And the above two points can be amplified when we use bandwidth-consuming demand tasks such as constant sum allocations.
Failure to read carefully from one conjoint card to the next is not a good thing, because it can lead to precisely the kinds of nonsense findings that we described above. More deeply, failure to comprehend the articulated benefits of one TPP variation versus another means that marketers are not getting any kind of read on their core question. If the participant does not notice that TPP#5 is qualitatively superior to TPP#6, your research is not doing its job.
And the data quality issues are not limited to how participants are orienting to the study stimuli. They also play out in the demand exercises – and particularly constant sum allocations, where the tendency to give sequences of share estimates that are rounded to the nearest 5 or 10 becomes overwhelming. My favorite example of this comes from a 2010 study that featured a retrospective analysis of rounded responses in a longitudinal study of personal health featuring nearly 18,000 participants.13 The researchers found that across nearly 40 different questions that involved numerical assessment, between 70% and 85% of the answers were rounded to multiple of 5 or 10 or were rounded to 0. You can find plenty of evidence in the academic literature about the extent of rounding in such exercises14, so I won’t belabor it here.
A Life Science-Appropriate Solution: Self-Explicated Conjoint
I am not a fan of pointing out problems with one approach unless I can specifically point to a better option, so we’ll close on a positive note with a high-quality alternative method. For our world of limited sample sizes and small effect sizes (to say nothing of limited budgets), we believe that self-explicated conjoints (SEC) are often a better choice than the experimentally-driven procedures in the conjoint family. We will be posting a complete account of the SEC method separately, so for the moment, I just want to cover the basics. In a self-explicated conjoint, your product profiles are pulled apart so that the participants can evaluate all of the product attributes relative to one another. They assign relative importance ratings to each attribute while seeing the entire list. Then, in a separate exercise, the rate the value that they ascribe to each level that comes along with each individual attribute, normally using something like a 1 to 10 scale. This simple two-step process gives you the same kinds of incremental, relative value data that you get from a derived partworth assessment in a traditional conjoint. These attribute/level values are easily integrated with demand estimates because they can be bounded by formal assessments of the best- and worst-case TPP variations.
While every tool in the methodological toolbox has limitations, we believe that SECs offer a nearly ideal option for a range of business reasons.
- They maximize the statistical power of your research, which is especially important when working in disease settings that have limited sample sizes. This stems from the fact that in an SEC (as compared with a blocked experimental condition from a conjoint or DCM), everybody responds to every possible attribute and level, rather than just seeing a tiny subset of all the possible combinations.
- They minimize the risk of nonsense findings because the customers are able to see the logical stepwise changes in attribute performance as you move from one level to another. This is precisely the kind of public task that elicits a need for cognitive consistency.15
- They are more affordable than studies using the conjoint family of methods because they are less intensive in setup and far less intensive in terms of analytic time.
- They take up less survey real estate than you would ordinarily have to use on a typical 8 to 10-card block design in a conjoint.
- Experimental comparisons between the findings from SEC studies compared with traditional conjoint family studies generally show very high convergent validity. They also show very high correspondence with real world choice outcomes and real world preference (i.e., nomological validity). 5,6,16,17,18
Some readers may regard the last bullet point with skepticism. A paper from the Journal of Marketing Research was published in 1997 that involved a head-to-head comparison of traditional full-profile conjoint against self-explicated conjoint to see which approach yielded greater predictive validity.18 The title tells you something about the cultural momentum that has grown up around the conjoint family: The Surprising Robustness of the Self-Explicated Approach to Customer Preference Structure Measurement. “Surprising” is the key word here. Why would it be surprising? At least in part it is due to the aforementioned mythology surrounding the idea of “revealed preference” being innately superior to stated preference, which we discussed earlier. However, that 1997 article is not alone – the bulk of the data suggest that SEC and traditional conjoints produce similar attribute importances.7 Further, a meta-analysis published all the way back in 2001 showed that, in 78% of such comparisons, the SEC data actually had better predictive validity vis-à-vis real-world outcomes compared to traditional conjoint.17 I want to be absolutely clear that I have read a handful of experimental comparisons that show authentic differences in attribute importances when using traditional conjoints compared with SEC, but these appear to be the exception not the rule. Given the totality of the evidence, I think that insights professionals can safely assume that SECs will give similar quality data to what you would get from the conjoint family in most situations.
Given the huge business advantages listed above, this option warrants serious consideration.
Wrap-Up & Take-Home Points
- The “conjoint family” of methods, including traditional conjoint and DCM designs have become nearly a default preference in the life science industry when it comes to evaluating the relative importance of product attributes, including in the context of demand studies.
- One common myth about these designs is that they carry a near-magic ability to reveal things about customer preferences that traditional direct-assessment methods cannot tell us, but that idea is not supported by scientific evidence.
- In the world of life science demand research, there is strong empirical evidence for three foundational problems with this family of methods.
- First, meta-analytic evidence suggests that conjoint methods may actually increase the degree of bias in customer preference estimates compared with self-stated methods.
- Second, conjoint methods have a tendency to produce what we call “nonsense findings” – where demand for an inferior TPP will be higher than demand for a superior TPP – and this tendency is amplified in life science research where we struggle with both small sample sizes and small effect sizes.
- Third, the use of repetitive card-based exercises in conjoint studies produces data quality issues relating to cognitive fatigue and flagging attention; these issues are amplified by the use of constant sum allocation exercises in demand studies.
- We believe that the Self-Explicated Conjoint method (SEC) represents a highly viable alternative for use in life science studies, and particularly in demand research, which remediates most of the challenges with the conjoint family and provides a variety of business-relevant advantages.
To learn more, contact us at info@euplexus.com.
About euPlexus
We are a team of life science insights veterans dedicated to amplifying life science marketing through evidence-based tools. One of our core values is to bring integrated, up-to-date perspectives on marketing-relevant science to our clients and the broader industry.
References
1 Rao, V. R. (2014). Applied conjoint analysis (No. 2014). New York: Springer.
2 Stefanelli, A., & Lukac, M. (2020). Subjects, trials, and levels: Statistical power in conjoint experiments.
3 Arazy, O., Kopak, R., & Hadar, I. (2017). Heuristic principles and differential judgments in the assessment of information quality. Journal of the Association for Information Systems, 18(5), 1.
4 Netzer, O., Toubia, O., Bradlow, E. T., Dahan, E., Evgeniou, T., Feinberg, F. M., … & Rao, V. R. (2008). Beyond conjoint analysis: Advances in preference measurement. Marketing Letters, 19, 337-354.
5 Choi, P. (2005). Conjoint Analysis: Data Quality Control. Wharton Research Scholars Journal, 4, 1.
6 Louviere, J. J., & Islam, T. (2008). A comparison of importance weights and willingness-to-pay measures derived from choice-based conjoint, constant sum scales and best–worst scaling. Journal of Business Research, 61(9), 903-911.
7 Van Ittersum, K., Pennings, J. M., Wansink, B., & Van Trijp, H. C. (2007). The validity of attribute-importance measurement: A review. Journal of Business Research, 60(11), 1177-1190.
8 Schmidt, J., & Bijmolt, T. H. (2020). Accurately measuring willingness to pay for consumer goods: a meta-analysis of the hypothetical bias. Journal of the Academy of Marketing Science, 48, 499-518.
9 Selka, S., Baier, D., & Kurz, P. (2013). The validity of conjoint analysis: An investigation of commercial studies over time. In Data analysis, machine learning and knowledge discovery (pp. 227-234). Cham: Springer International Publishing.
10 Serdar, C. C., Cihan, M., Yücel, D., & Serdar, M. A. (2021). Sample size, power and effect size revisited: simplified and practical approaches in pre-clinical, clinical and laboratory studies. Biochemia medica, 31(1), 27-53.
11 Jeong, D., Aggarwal, S., Robinson, J., Kumar, N., Spearot, A., & Park, D. S. (2023). Exhaustive or exhausting? Evidence on respondent fatigue in long surveys. Journal of Development Economics, 161, 102992.
12 Toubia, O. (2018). Conjoint analysis. Handbook of marketing analytics: Methods and applications in marketing management, public policy, and litigation support, 59-75.
13 Manski, C. F., & Molinari, F. (2010). Rounding probabilistic expectations in surveys. Journal of Business & Economic Statistics, 28(2), 219-231.
14 Schnell, R., Redlich, S., & Göritz, A. S. (2022). Conditional Pop-up Reminders Reduce Incidence of Rounding in Web Surveys. Field Methods, 34(4), 334-345.
15 Van Kampen, H. S. (2019). The principle of consistency and the cause and function of behaviour. Behavioural processes, 159, 42-54.
16 Surana, A. (2024). Comparative analysis of conjoint analysis methods: Full profile vs. self-explicated approaches. IFSMRC African international Journal of Research in Management, 12(03), 01-06.
17 Sattler, H., & Hensel-Börner, S. (2001). A comparison of conjoint measurement with self-explicated approaches (pp. 121-133). Springer Berlin Heidelberg.
18 Srinivasan, V., & Park, C. S. (1997). Surprising robustness of the self-explicated approach to customer preference structure measurement. Journal of Marketing Research, 34(2), 286-291.